A Simulator for Marine Litter Observation from Space
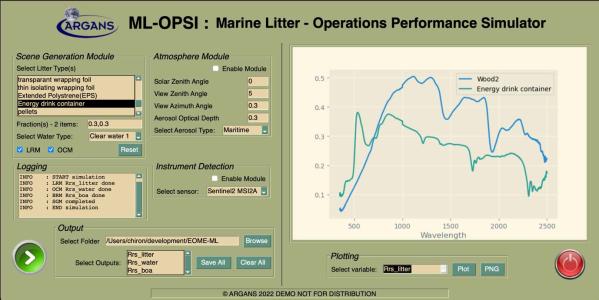
“Remote Sensing for Marine Litter - RESMALI” has identified the ideal parameters to study marine litter (ML) from space, including spectral bands with affordable signal-to-noise ratio (SNR) at top-of-atmosphere (TOA) level, in a range of wavelengths from visible to SWIR. To continue this path towards a dedicated mission, performance assessment against simulated real-case scenarios of ML is key. We want to build a simulator to estimate ML signal at TOA from a set of scenarios representing the various applications already targeted by the community (e.g. windrows, frontal areas, river mouths, sub-tropical gyres). The simulator will have two components: (1) An scenario builder based on Sentinel-2 data, to be artificially “contaminated” by ML, and with super resolution skills; (2) a radiative transfer model from ocean’s surface to TOA able of coping with varying atmospheric conditions and observational geometries. The simulator will consider the changes ML causes in the water reflectance, exploiting gathered information of plastic polymers (already provided in RESMALI). Different, viewing geometries, aerosol models and cloud conditions can also be considered, as naturally occurring in the Sentinel-2 data. ML scenarios of reference will be built based on in-situ campaigns, to reflect the true littering conditions at each case, both in spatial distribution and composition. The simulator will be validated over artificial targets at sea in a field campaign including spectral measurements from ASD, on-field radiometers, and cameras on UAVs, concomitant with Sentinel-2 acquisitions. Combined, they can be used to estimate atmospheric contribution and assess performance of the simulator. The expected outcomes will be: (1) an improved definition of the future instrument, including optimized selection of spectral bands; (2) a retrieval multispectral algorithm able to detect and quantify ML presence; (3) a set of case studies that could be used as benchmark for future developments