Analysis of Flight Data using Model based driven diagnostics
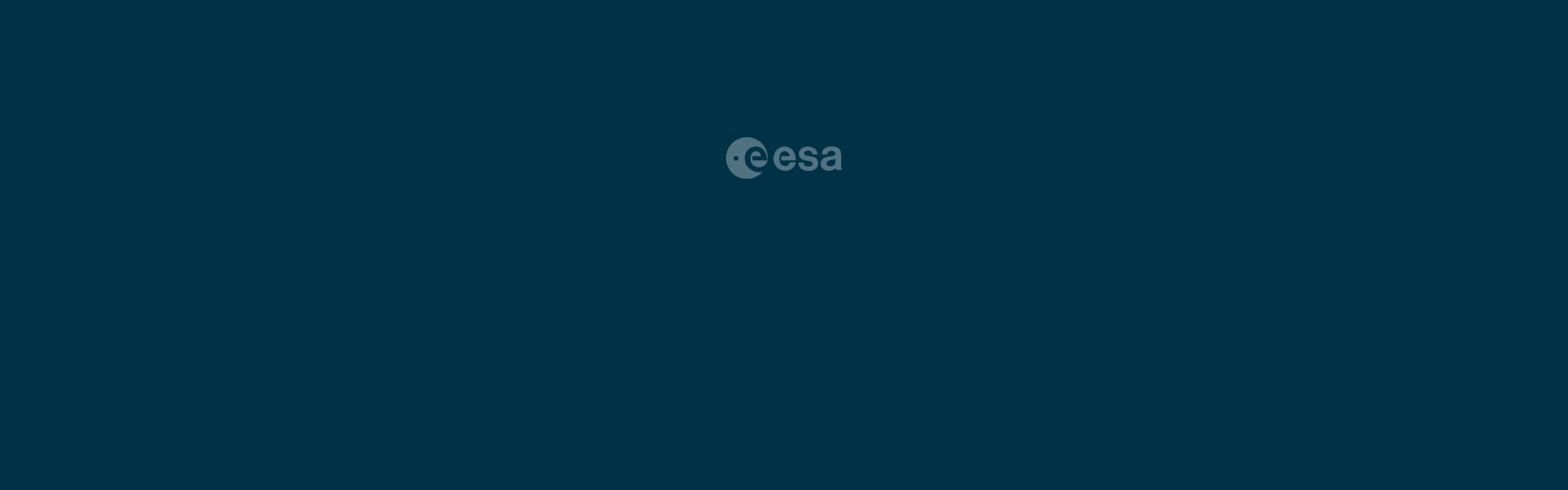
This activity aims at developing and prototyping a methodology based on model-based planning that allows diagnostic and refinement of spacecraft discipline models. This activity will allow better spacecraft health monitoring, to anticipate and avoid anomalies or to solve anomalies more efficiently. In addition it improves the feedback chain from flight to design and verification engineers, and fosters collaboration between operations and spacecraft design engineers.
Efforts for anomaly root cause analysis (e.g. GAIA) show the urgent need of using discipline models with flight data for diagnostic purposes. Acquiring more feedback from (also nominal) flight operations is also urgent to improve spacecraft related processes of engineering, analysis and verification. A spacecraft is equipped with several monitoring sensors that measure different attributes of the spacecraft. This results in a huge raw stream of data that has to be analysed to produce information about spacecraft behaviour and health status. In the past different diagnostic tools/algorithms have been introduced to support domain experts in their daily work. The innovative idea of the study is to introduce AI planning functionality, based on discipline models of spacecraft subsystems, to support and further enhance the diagnostic analysis of big data volume (as it can be the set of data produced by a spacecraft along its mission life) as well as the refinement and update of spacecraft discipline models. This functionality shall be included in a software framework which encompasses the algorithms necessary to deal with the evolution of the discipline models over the different mission phases. Two main expected results are respectively to improve the capability of identifying anomaly root causes and to support the definition and/or refinement of the discipline models of spacecraft subsystems. In addition, better analysis of flight data and behavioural prediction (e.g. what-if analysis) will be enabled. Given a specific status of the spacecraft (for instance this can be the case of anomaly situation), the expected system shall use AI model-based planning to generate plausible and consistent flow of actions (i.e., plans) that have brought the S/C in such a particular status. These plans can be seen as hypotheses that justify a particular behaviour of the S/C. Planning-based reasoning is enabled by discipline models which describes entities in the world, their states, and relationship to observations. The hypotheses identified by the planner are then verified against available data (this requires to create a mapping between model parameters and telemetry parameters). This step will prune out all the not consistent hypothesis, leading to a more accurate and direct analysis of the different combinations of parameters. When a consistent plan (hypothesis) does not exist, then the discipline model is recognized as not complete or 'wrong'. This case will trigger further analysis to identify flaws in the discipline model and eventually refine the model (either automatically or manually). This study requires the possibility to use discipline models with flight data and the necessary developments (specification / data mapping) are part of the study. Furthermore the proposed AI Planning Scheduling technology has been already mastered in other GSP and TRP studies to design, prototype and validated with success advanced mission planning systems for spacecraft and robotic operations.