Hybrid Edge-Cloud AI Accelerated Astrometric Reduction Pipeline for Agile Near-Real Time In-Situ Space Surveillance and Tracking
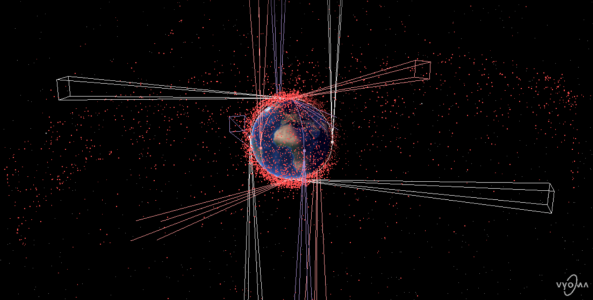
With the 'democratisation of space', and the proliferation of large non-geostationary orbit constellations, the pace at which human-made objects are being deployed into orbit demands urgent action in defining and supporting a global space traffic management plan.
Promptly identifying, tracking, and cataloguing resident space objects, in particular after orbit insertion or break-up and fragmentation events, as the recent Russian anti-satellite weapon test put in evidence, is of critical importance to enable the safe and sustainable use of space. However, the current installed ground-based space surveillance and tracking (SST) capacity might not be sufficient to cope the expected exponential growth in RSO population in the coming decade, due to the intrinsic limitations of current tracking techniques.
One very promising approach to address this issue without the logistic hurdles and costly operations of a wider ground capacity, is space-based space surveillance (SBSS). The concept of optical SBSS is not new, and different missions, like the Canadian Space Agency’s Sapphire and NEOSSat among others, have proven the concept and validated its technological readiness. However, their mission designs have focused on the instrument in-orbit validation and provision of tasked tracking function, relying entirely on ground-based post-processing of the images captured on board, non-suitable for large-scale SST.
This study aimed precisely at accelerating the Technological Readiness Level of an agile and cost-effective next-generation SBSS mission concept, devised around a hybrid edge-cloud processing pipeline, supported by computer cision techniques for the different steps of the astrometric reduction and measurement formation chain, and commercial off-the-shelf hardware to accelerate machine learning models both in the edge (on board) and in the cloud (on ground).