Adaptive SAR signal compression through Artificial Intelligence
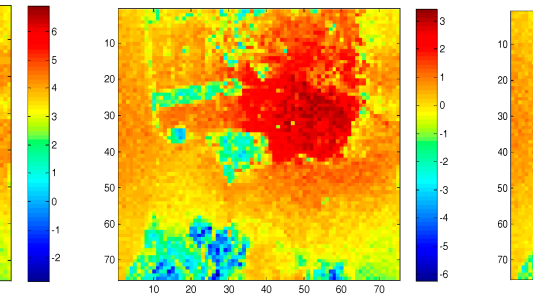
This project was primarily focused on exploring and enhancing the state-of-the-art in raw Synthetic Aperture Radar (SAR) data compression by incorporating machine learning (ML) techniques. The overarching goal was to evaluate the potential improvements in compression efficacy through the integration of ML algorithms with the aim to on-board these algorithms in future. The project began with an extensive analysis of existing raw SAR datasets and current compression algorithms, including the standard Block Adaptive Quantization (BAQ) and its more advanced iterations like Flexible BAQ, Entropy Constrained BAQ, and FFT-BAQ. While these evolutions offer improved performance over standard BAQ for the same bitrates, they also introduce greater complexity. A key innovation proposed in this project was the concept of “local optimum” SAR data compression. This approach adapts compression to the specific scenarios observed by the radar instrument, leveraging machine learning for enhanced dynamism and feature identification in the received signals. The ML algorithms enable on-board identification of areas of the raw data that can be compressed with lossier, less complex algorithms whilst still meeting a chosen reconstruction error threshold. This facilitates the selection of the most effective compression scheme tailored to the unique characteristics of each data set. The initial phase of the project involved a comprehensive survey of publicly available raw SAR datasets and simulators, which informed the selection of relevant applications and frequency bandwidths for detailed study. Subsequently, a thorough analysis and trade-off of current SAR compression algorithms were conducted. This process involved evaluating these algorithms against various metrics such as applicability to specific bands/applications and complexity measures. The project then moved to assess the feasibility and capabilities of AI/ML in this context. A desktop prototype was developed that could select algorithms based on statistical features of the raw data and compress different sets of range lines with different algorithms through the scene. Finally, there was an examination of hardware requirements and constraints. This was crucial in understanding the practical aspects of implementing ML-based compression algorithms in real-world SAR applications.