Robust machine learning systems for dependable space applications
Programme
GSTP
Programme Reference
GT1I-306ED
Prime Contractor
e:fs TechHub GmbH
Start Date
End Date
Status
Closed
Country
Germany
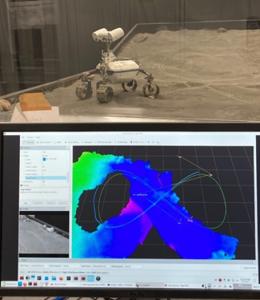
Objectives
Objective of this activity is to develop methods for detection and rejection of adversarial examples in security sensitive and safety-critical systems using deep Convolutional Neural Networks (CNNs). This is an essential activity to enable use of Artificial Intelligence (AI) and Machine Learning (ML) on the edge on mission critical applications.
Description
;;
Recent developments in AI and Machine learning research have proven that many different models of inference system have potential use in next generation space missions for on-board data analysis; autonomous decision making; sensor diagnostic and prognostic; etc. These methods are highly flexible computational tools supported by energy efficient hardware accelerators. There is still an unsolved problem to explain the relationships between their inputs and their final outputs, especially on AI systems based on deep learning techniques.
;
The inability to backtrack the inference process in a meaningful way hinders the adoption of AI models for safety-critical systems which require the inference process to be manifest and non-ambiguous, possibly supported by well defined mathematical models. Machine learning systems are not robust by default. Even systems that outperform humans in a particular domain can fail at solving simple problems if subtle differences are introduced.
;
For dependable systems we need a solution that evaluates the AI/ML network as a black box algorithm already trained to perform a specific task, and evaluates the 'corner cases' in (for example) image analysis. The idea is to develop a modular framework that can be adopted for multiple analyses. We need to supplement next AI inference systems with a set of operational conditions and ranges of tolerance for user-defined input distortions that may serve as a first step in defining the robustness of the system to errors and faults, possibly bridging the gap between safety-critical systems and AI systems towards the adoption of AI engines in future space applications.
;
The core this activity is on the set-up of a full end-to-end tool-chain and neural-network inference framework that can be trained on-ground (e.g. cloud based) and then be deployed and executed on a typical flight system, with particular care on fault tolerant techniques and verification and validation problems, impacting use of AI/ML in all relevant space missions.
Tasks:
-
- Definition of ML use cases in mission critical applications (example rover guidance, star tracking, FDIR management, autonomous collision avoidance manoeuvres);
- Definition of target hardware (Accelerators, FPGA, processors) and of suitable FT techniques to be adopted;
- Development of an Engineering Model, using representative space graded HW.
- Verification and validation of the developed EM.
;
- ;Verification and validation of the developed EM.
- ;
;
Application Domain
GEN-Generic Technologies
Technology Domain
1-On-board Data Subsystems
2-Space System Software
8-System Design & Verification
Competence Domain
3-Avionic Systems
Keywords
49-Artificial Intelligence
Initial TRL
TRL 3
Target TRL
TRL 5
Achieved TRL
TRL 5
Public Document
Executive Summary
Final Presentation