Detection of Ocean Litter Plastics with Hyper-to-multispectral Infrared Neural Networks (DOLPHINN)
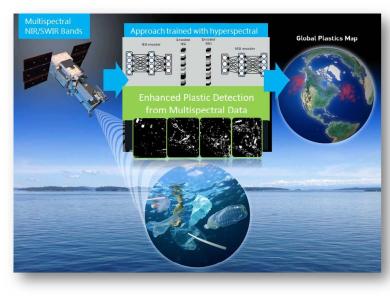
Successfully mitigating the damage caused by marine litter requires large-scale, accurate mapping of oceanborne plastics across the globe. Quantifying where and how much plastic debris enters the marine environment in a global spatio-temporal sense is critical in formulating solutions to the plastic pollution problem. Satellite-based measurements can help to survey remote, isolated locations in which direct observations are sparse, costly, and difficult. Plastics commonly found as ocean litter have spectral features that can be detected with multispectral assets such as Sentinel-2, but the sensor’s diminished ability to distinguish between plastic and other material types makes it difficult to create reliable detection maps. Hyperspectral sensors, such as the upcoming CHIME mission, have better spectral resolution that allows for more successful plastic detection, but there are few currently available spaceborne HSI sensors.
The objective of our study is to develop and assess the feasibility of an enhanced multispectral plastic detection algorithm, leveraging the latest in machine learning approaches. We develop a novel neural network called Joint Plastic Spectral Embedding (JPSEmbed) that learns to associate multispectral data with the more capable hyperspectral data in a way that allows for better detection of plastic when using only multispectral input. Multispectral sensors on orbit can then better contribute to the creation of plastic detection and concentration maps along with hyperspectral ones as they become available.
In testing JPSEmbed with both simulated and real Sentinel-2 data, we successfully detect land-based plastics significantly better than a traditional multispectral detector (Maximum Likelihood) and demonstrate we can detect known plastic land targets such as greenhouses. We demonstrate the ability to tailor training to reduce the spurious false detections in the scene, and that adding more spectral bands has the potential to further increase performance. When we modify the approach to detect concentration of plastics as fractions of pixels, we find the method promising with initial concentrations reported begin within a 20% error 72% of the time.
Future work to mature the approach to operationally-ready performance for shoreline/land plastics using real Sentinel-2 data can provide key information about where land plastics are making their way into waterways. Expanding the approach to other sensor types would allow for more widespread coverage and more frequent updates of plastic detection and concentration maps. Accounting for factors specific to detect floating marine debris would provide full global plastic detection and concentration maps combined from a variety of space-borne multispectral sensors offering large-scale tracking for clean-up and mitigation efforts.