Remote sensing for marine litter – Early Technology Development Scheme (REACT)
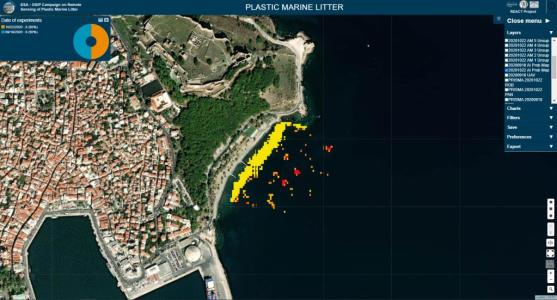
The main objectives of the REACT project were to:
▪ Assess how plastic litter can be detected and quantified with current and future remote sensing tools;
▪ Develop adaptive indices insensible to biases induced by sunglint on satellite radiometric products and indicate the constraints of current satellite missions under various atmospheric and illumination conditions;
▪ Exploit data fusion methods between remote sensing data with high spectral resolution (PRISMA hyperspectral, Sentinel-2) and high spatial resolution (PRISMA panchromatic, WorldView) to increase the sensors’ detectability of marine plastic litter;
▪ Explore spectral unmixing methodology for sub-pixel detection of floating marine plastic debris;
▪ Explore artificial intelligence techniques for detecting plastic litter;
▪ Conduct controlled experiments under real-life conditions to better understand the effect of the atmosphere and the illumination conditions on the spectral properties of marine plastic in visible and infrared wavelengths.
During the project, a few controlled experiments were realised to understand better the effect of the atmosphere and the illumination conditions on the spectral properties of marine plastics in visible and infrared wavelengths. Twelve floating plastic targets were constructed for the experiment needs. Their sizes were selected according to the spatial resolution of data expected to be achieved by image fusion. Targets were realised with four types/compositions of plastic materials with various colours: 1) low-density polyethylene (tarps in white, yellow and green colour), 2) polyethylene terephthalate (transparent water bottles, green oil bottles), 3) polystyrene (sheets for building insulation in cyan colour), and 4) all the above materials in equal surface extent. The 12 targets were placed offshore and onshore during satellite data collection. In-situ measurements using the spectro-radiometer were also carried out during the controlled experiments. The controlled experiments were realised in Mytilini and Koplos Geras, on the Greek island of Lesvos.
The MS and HS satellite imagery collected during the controlled experiments were applied image fusion techniques to increase the information content of the resulting merged images. More in detail, Spectral Signature Unmixing techniques and Artificial Intelligence algorithms were used.
Spectral Signature Unmixing contributes to the extraction of information at the sub-pixel level. Its main scope is to detect the distinct spectra in the fused MS and HS scenes, which may represent materials, and to estimate their apparent quantification in a pixel in terms of a fraction. Endmembers, or pure pixels, correspond to these different signals. In contrast, abundances refer to the fractions of these endmembers within a mixed pixel. This project achieved marine plastic litter detection by separating endmember spectral that best characterise plastic materials and waters. The abundance maps led to plastic targets detection.
Apart from Spectral Signature Unmixing, plastic targets were also detected by supervised and unsupervised ML algorithms. The output was probability maps representing the probability that a pixel contains plastic or not.
The main findings of the project are summarised below:
▪ Plastic and water spectral signatures are significantly correlated in the original images and the fused HS data. The Principle Component Analysis (PCA) and the Gram-Schmidt Adaptive gave better results in terms of spectral discrimination between water and plastics.
▪ Sunglint correction provided no beneficial in spectral discrimination between water and plastics; thus, it is not necessary for pre-processing
▪ Fusion image based on matrix factorisation performed better than the deep learning methods. CNMF method produced better results when plastic targets were placed offshore. On the other hand, the HySure method presented slightly better results with targets placed onshore.
▪ SSU was able to detect plastic targets, although some manual tuning is necessary. Same for plastic detection through some plastic indexes.
▪ AI algorithms provided promising results in plastic detection, although with a small dataset.
▪ Land and swallow waters masking is required by both SSU and AI
▪ No significant results were highlighted with the targets onshore with both SSU and AI.
Abundance maps and probability maps represent a valuable tool to support ARPA for the monitoring activities of the Descriptors 10 of the MSFD. The output of REACT can support the authorities (Environmental Agencies, Universities, Research Centres) responsible for the monitoring. In particular, maps of abundance and probability can help define monitoring plans to evaluate the optimal position for a monitoring station.
In addition, maps of the spatial and temporal distribution of marine litter can be used in modelling the dispersal of plastic litter in aquatic systems from local to global scales. Indeed, the application of current data from remote sensing via satellite can become an efficient and reliable tool to monitor large marine areas.