for In-Flight artificial Intelligence Proof of Concept Experiments
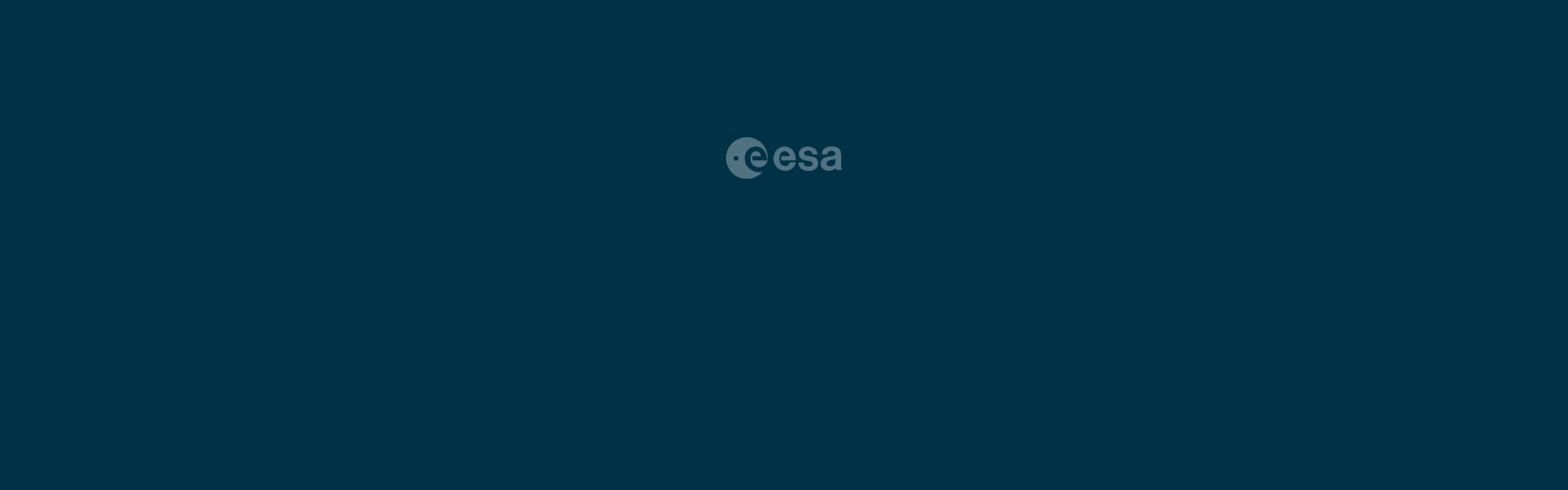
Activity objective is to assess Myriad-2 performance in space for typical platform and payload video processing applications. The proposed IOD/ICE Cube activities have several objectives: 1. Support for IOD on Tyvak?s Mark I with Hyperscout-2 cubesat. Cubesat will fly a Myriad-2 board to perform in orbit data processing of hyperspectral data. - This in collaboration with EOP-8, EOP-P and ESRIN and will utilize EOP state-of-the-art data and knowledge for development of deep learning applications demonstrators. - This is a very important capability demonstration and de-risk activity (the first of this kind), since it will allow to demonstrate in space usefulness of having L1 and L2 data produced and capability of data downselection and autonomous end-user product generation. From technological point of view, a great deal of information will be gathered on the in orbit performance of the Myriad-2 chip and the basic SW radiation countermeasures implemented. - Current AI Miryad2 board is already TRL 5 and can fully support IOD on Hyperscout-2 mission. Radiation tests performed have fully demonstrated suitability of proposed approach. - Data processing from this activity will help to develop a stable operational end to end approach for future AI-powered EO cubesat missions. 2. ISS space cube IOD (inside the station). Myriad-2 board will be used to perform House Keeping Telemetry (HKTM) analysis of a set of miniaturized sensors (temperature, position, accelerometers, IMU, and FDIR simulation) - This demo activity may open a door for future autonomous FDIR management of functional redundant sensors/actuators in complex systems in complex environments. 3. ISS space cube IOD (external location on ISS). A computer vision package (stereo camera, grayscale camera, IMU, radiation dosimeter will be deployed and used to test edge computer vision applications on the field (feature extraction, debris monitoring, pose estimation, star/earth/moon tracking). - This is a fundamental demonstrator for all future RDV-Docking and e.deorbit minisatellite missions. - Activity will produce a ready to use hardware for ISS cube IOD 4. On board processing for vessel recognition (Norwegian Minisatellite - SPIRE) - Data fusion with in orbit AIS data will allow use of Myriad-2 board to perform: - Vessel detection (in the narrow sense): Finding vessel candidates in the image and locating them; - Vessel classification: discriminating detected targets between vessel/non-vessel and then getting the class of the vessel (e.g. Fishing, tanker, cargo); and - Vessel identification: establishing the unique identity of the vessel (e.g. International Maritime Organization (IMO) number, Maritime Mobile Service Identity (MMSI) number, name), in integration with on board AIS receivers.
The preparation tasks for the IOD activities can be grouped as follows: 1. Procurement of a set of standard cubesat-grade HW board hosting Myriad-2 accelerator chips (building up on test breadboard developed in GSTP de-risk activity). Boards will be equipped with a rad-tolerant voltage regulator, with a latching current limiter for restartable latch up protection, and with ethernet interface.A mass production (several 10s of boards) can be initiated, to support also destructive reliability/robustness test activities. 2. Board level safety and robustness tests of the boards (TVAC, radiation, vibration, conformal coating) for the IOD flights. 3. Set up of a dedicated deep learning infrastructureDeveloping deep neural networks involves unique requirements that fundamentally change ?classical? SW development process. These include:a)Specialized hardware, generally in the form of graphics processing units (GPUs), tensor chips (TPUs) or high performance FPGAs in serversb)Storage for massive training and test datasets that only continue to growc)High throughput I/O between this specialized hardware and high volume storaged)The SW backbone that will be used for DNN learning and inference preparation is already available (tensorflow, Caffe, OpenVINO) and all released under open source licenses (either GPL or Apache).