Intelligent Operations and Preventative Maintenance Assistant
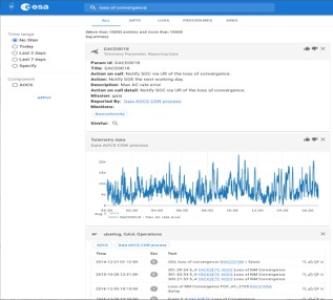
Prototype an operations assistant utilising pattern detection, event processing, machine learning techniques to improve efficiency, predict failure and enable novel operations / maintenance concepts.
An Operations Ground Segment consists of a complex system of systems, each of which is a source of high-throughput events e.g. telemetry, Out-Of-Limits, operating/data/network system logs. Today the majority of MC systems support a particular subsystem, have limited processing / analysis capabilities (e.g. limit checking, synthetic parameters, filters) and support only stateless operations. Existing analysis tools for data analytics and Machine Learning (ML) techniques are regulated to mission archive data rather than on online systems and data streams.Manual operator instructions/workarounds based on events are maintained and evolved throughout a mission, e.g. in an access database. These are exacerbated by increasing data rates, mission complexity and fewer operators per mission. Recent studies have assessed the application of Complex Event Processing (CEP) techniques to build a source-agnostic, adaptable and automated monitoring capability. This enables correlation and pattern-based detection at system level, acting on real-time events and alerting the operator. Operators program their knowledge into engine rules. Use case examples include correlation of star tracker (ST) blinding with planning, or root-cause of loss of AOCS convergence based on environmental/operational conditions.Further studies have assessed the use of ML techniques for predictive analytics and preventative maintenance concepts for mission data systems. This activity seeks to combine these two technologies and lessons learned to develop an intelligent operations assistant, capable of detection (e.g. via CEP rules or ML patterns), proposal of subsequent preventative / corrective actions, through to their execution. As simple example, to automatically ignore a ST blinding event or to switch to a redundant ST. The prototype shall demonstrate a proof of concept based on a representative use case, interfacing with the real mission data systems. The activity encompasses the following tasks: - Analyze results and outputs of previous CEP and ML studies- Define use cases for both mission operations and preventative system maintenance which combine the use of event stream analytics and AI-driven reasoning- Trade-off extension / integration options for previously developed tools and define the high-level architecture of an integrated solution- Develop a prototype of the intelligent assistant tool- Demonstrate use cases and identify lessons learned and required future development work