Prototype Retrieval Algorithms Based on Synergistic Use of Microwave and Optical Imagers for land application
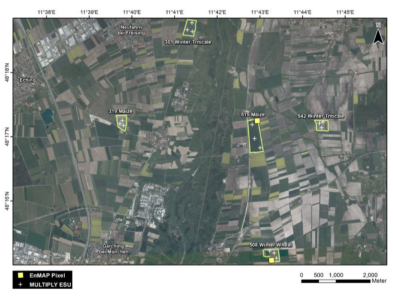
With the launch of the first Sentinel satellites, a new era in Earth observation has started that allows for the development of new approaches that use these novel observations at high temporal frequencies and fine spatial resolution with complementary information from the optical and microwave spectral domains. One very important application for is the monitoring of crops.
Optical remote sensing of crops and crop yields has a long history, stretching back as nearly far as the advent of satellite remote sensing itself (e.g. Idso et al. 1977, Tucker 1980). Most early examples use vegetation indices (primarily NDVI — the Normalised Difference Reflectance Index) from AVHRR or Landsat data and calibrate empirical relationships with variables such as yield or leaf area. Indeed, even today the majority of studies using optical remote sensing data to monitor crops continue to focus on the use of vegetation indices. For example, NDVI for yield determination in soybean (Liu and Kogan, 2002), PVI for determining faPAR in corn (Weigand et al. 1986), LAI from RVI in maize (Gardber and Blad, 1986) and TSAVI for determining green crop area in wheat (Broge and Mortensen, 2002). Vina et al. (2011) compared a large number of vegetation indices to estimate the leaf area index of maize and soybean and found that those designed to be sensitive to chlorophyll concentration (e.g. the MERIS Terrestrial Chlorophyll Index, MTCI) gave the best predictions. The advantage of these and similar techniques is that they are relatively straightforward to apply and computationally cheap to implement. In principle they give reasonable results for the areas and cover types over which they were calibrated. However, their major shortcoming is that with little or no underlying physics they are not generalizable beyond the areas which they were calibrated and applications on an appreciable scale are unlikely to yield accurate results. In particular such techniques are not amenable to combining data from different sensors and different domains of the electromagnetic spectrum. If we want to combine data from optical sensors such as Sentinel-2 with SAR sensors such as Sentinel-1 some form of physical-based model will be required.