Task-driven super-resolution of Sentinel-2 images
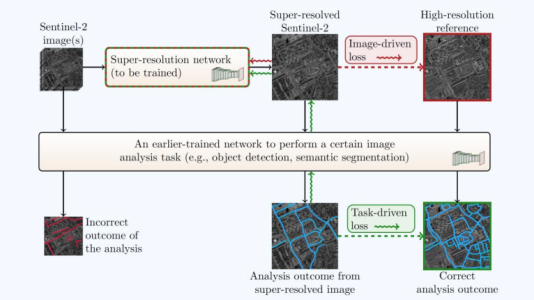
Super-resolution (SR) is aimed at reconstructing high-resolution (HR) images from low-resolution (LR) observations. Developing effective SR techniques is particularly important for enhancing the capabilities of remotely-sensed data, including Sentinel-2 images, as their 10m resolution remains a serious limitation in many cases. Importantly, most of the state-of-the-art SR techniques based on deep networks are evaluated in an artificial setup: LR images are simulated from an HR image (later treated as a reference), and the goal of SR is to reverse this degradation process. As the degradation model commonly does not reflect the actual LR-to-HR relation, this leads to over-optimistic SR performance for simulated data which drops significantly for real-world images (i.e., not artificially degraded). The recently-published datasets that match Sentinel-2 and HR images do not solve this problem entirely - LR and HR images vary significantly due to different acquisition conditions, which makes it challenging to rely on image similarity-based loss functions during training. In this project, we will exploit high-level image analysis tasks, such as detecting road networks, for training SR models in a self-supervised manner. We will propose new loss functions based on existing image analysis deep models, which will guide the training so that the SR outcome leads to a similar analysis result as that obtained from the HR image, thus ensuring the practical value of SR. Importantly, the reference data will be generated automatically by processing HR images with the image analysis models, which will help avoid a tedious manual image annotation process. Although it was proposed to evaluate Sentinel-2 SR in application-related scenarios, the latter were not exploited actively to guide the SR training.
We expect that the proposed framework will lead to obtaining a new quality of super-resolved Sentinel-2 products that will be suitable for automated image analysis.