Deep Learning for Space
Programme
TDE
Programme Reference
T708-609OS
Prime Contractor
Solenix Engineering GmbH
Start Date
End Date
Status
Closed
Country
Germany
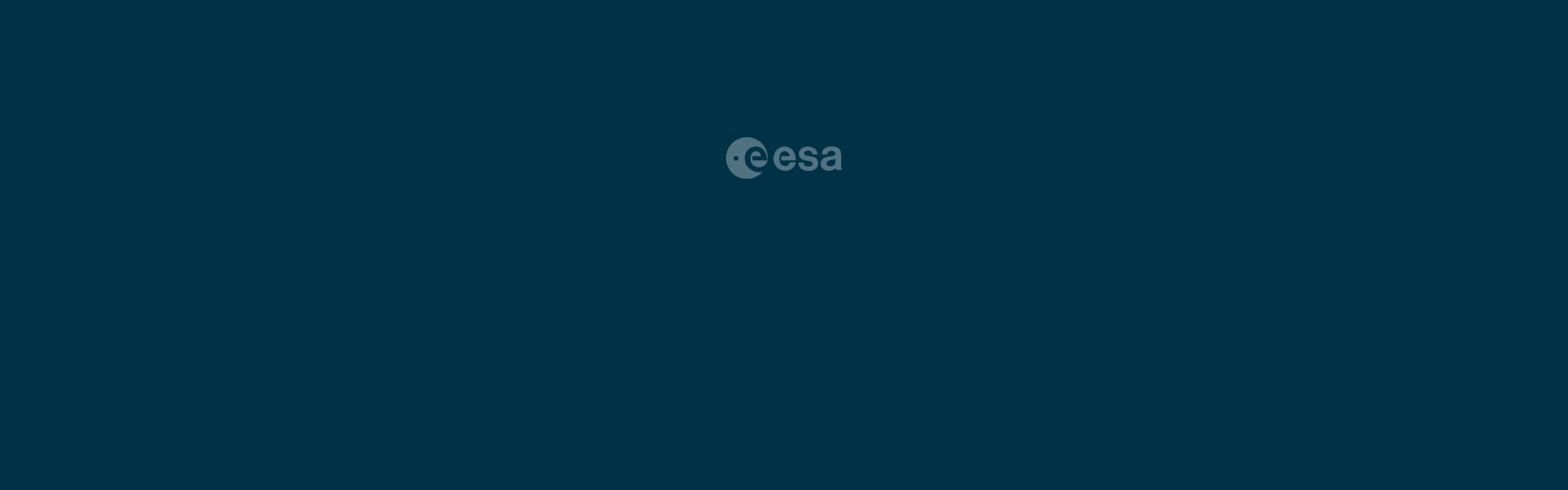
Objectives
Explore deep learning methods to assess the applicability and impact (new features, cost reduction, risk mitigation) of using deep learning techniques and models to space applications
Description
The activity foresee the assessment of several different deep learning architectures and deep learning algorithms. Initial use cases, for evaluation, will be selected from Operations and AIT data, supporting tasks such as spacecraft performance assessment, diagnostics and forecasting.
Thanks to newly available powerful cpus and gpus the Deep Learning technology is becoming one of the new emerging machine learning techniques capable to extract information and knowledge, mainly applied to visual related data, such as image classification. This activity is focused on the applicability of deep learning on non visual data, such as time series big data from space activities, investigating its potential added value for, e.g. Performance assessment, anomaly detection, diagnostics and forecasting, as well as behavioural modelling.
This activity encompasses the following tasks:
- Collect and select a suite of use cases from Operations and AIT, based on their level of maturity and availability of quality data
- Analyse existing deep learning techniques and preselect the most promising techniques suitable to be applied to time series data
- Implement the selected artificial neural network architectures for deep learning evaluation
- Perform trial and error implementation and assessment of several algorithms applied to the selected use cases
- Evaluate and assess the different deep learning algorithms and their impact in solving the proposed use cases
- Compile recommendations and guidelines for future development of deep learning operational applications for space
;
This activity provides an in-depth first evaluation of the promising deep learning technique applied to space data (excluding images). Its output, based on concrete use cases from Operations and AIT will pave the way towards the
exploitation in the coming future of deep learning to enhance the performance and reduce cost of activities, such as performance assessment, diagnostics and forecasting applied to the final phase of spacecraft development (AIT) and to operations. A guideline will be provided to speed up dissemination of deep learning technology adoption.
;
Software shall be delivered under an ESA Software Community Licence, so that any individuals or entities within ESA Member States can access to it and can provide update to the community of users.
Application Domain
Generic Technologies
Competence Domain
9-Digital Engineering
Initial TRL
TRL 2
Target TRL
TRL 4
Achieved TRL
TRL 3
Public Document
Final Presentation
Executive Summary